Project Topics
On this page, we provide the current project topics we offer. The project topics can be adapted for Diploma Theses, Master Theses, Bachelor Theses, Research Projects, Student Reports (Fachstudien), and Study Projects. It is also possible to submit your own proposal for a project.
Interested in our project topics or you have your own proposal? Please send your inquiry to scsp@iaas.uni-stuttgart.de.
When sending the inquiry, please consider the following:
- You are a student enrolled in a study program offered by or associated with the Computer Science department.
- The inquiry includes one or more of the topics below.
- In the case of your own proposal, describe the topic and its relevance to our research interests.
We receive many inquiries, so we ask for your patience in waiting for an answer. If you do not hear from us within two weeks, please send a reminder to the same email address.
We are looking for a student interested in technology transfer for which funds are available. Please contact Prof. Aiello if you are interested in exploring this opportunity.
Modeling the Behavior of Platoons of Motorcyclists Driving in the Black Forest
In 1992, Nagel and Schreckenberg presented a cellular model of traffic that with just four rules describes quite well highway traffic behavior. In the proposed project, the idea is to research a similar model for motorcycle platooning. Starting from maps of winding roads of the black forest, the goal is to build a cellular automata model of a platoon of motorcycles driving through those roads; i.e., to model the behaviour of a group of motorcyclists that are driving together without passing each other.
Possible metrics for evaluation are the acceleration/deceleration of vehicles depending on position in the platoon, how fun a road is based on the patter of curves and the size of the platoon and how often it is necessary to brake beacuse of a motorcycle in front, comparison with original car traffic model.
Literature:
[1] Nagel, K.; Schreckenberg, M. (1992). "A cellular automaton model for freeway traffic" (PDF). Journal de Physique I. 2 (12): 2221. Bibcode:1992JPhy1...2.2221N. doi:10.1051/jp1:1992277. Archived from the original (PDF) on 2014-03-11.
[2] Meng, J. P., Dai, S. Q., Dong, L. Y., & Zhang, J. F. (2007). Cellular automaton model for mixed traffic flow with motorcycles. Physica A: Statistical Mechanics and Its Applications, 380, 470-480.
[3] Lan, L. W., Chiou, Y. C., Lin, Z. S., & Hsu, C. C. (2010). Cellular automaton simulations for mixed traffic with erratic motorcycles’ behaviours. Physica A: Statistical Mechanics and its Applications, 389(10), 2077-2089.
[4] http://modelingcommons.org/browse/one_model/4806#model_tabs_browse_nlw
Supervisors: Prof. Dr. Marco Aiello
The Integration of Electric Vehicles in the Smart Grid
Electric Vehicles (eV) have a battery size that can vary from few KWh to almost 100 KWh. These are frequently connected to the power grid to charge. Several researchers have proposed the idea that these batteries can also be used to store grid electricity and provided it back to grid during peak demand periods. The research proposed here is to assess technical solutions to make the eV an integral part of the power grid, to study individual strategies based on dynamic pricing for incentivising eV integration, and to assess the economic viability of such approaches.
Literature
P. P. Malya, L. Fiorini, M. Rouhani, and M. Aiello (2021) Electric Vehicles as Distribution Grid Batteries: A Reality Check, Energy Informatics, Springer, 4(Suppl 2):2.
A. P. Lopes, F. J. Soares and P. M. R. Almeida, "Integration of Electric Vehicles in the Electric Power System," in Proceedings of the IEEE, vol. 99, no. 1, pp. 168-183, Jan. 2011.
Francis Mwasilu, Jackson John Justo, Eun-Kyung Kim, Ton Duc Do, Jin-Woo Jung, “Electric vehicles and smart grid interaction: A review on vehicle to grid and renewable energy sources integration,” in Renewable and Sustainable Energy Reviews, vol. 34, no. 6, pp 501-516, Jun. 2014.
Supervisor: Prof. Dr. Marco Aiello
On the energy accountability of running software
Software running on data centres and personal computers is responsible for a large amount of energy consumption and consequent emissions worldwide. At the same time, it is very difficult to perform an accurate measure of energy consumption for individual software program and its individual components, this is also due to the current software engineering practices of layering and virtualisation. For this research project you are asked to look into energy models for software, energy accountability for software, comparing the running of the same algorithm on different platforms for measuring the energy consumption, etc.
Literature
[1] S. Miller and C. Lerche (2022) Sustainability with Rust. AWS Open Source Blog. Online: https://aws.amazon.com/de/blogs/opensource/sustainability-with-rust/.
[2] Green Software Lab (2018) Energy Efficiency in Programming Languages (source code of 10 benchmarks implemented in 28 programming languages). GitHub. Online: https://github.com/greensoftwarelab/Energy-Languages.
Supervisor: Prof. Dr. Marco Aiello, Dr. Ilche Georgievski
LLM for AI Planning
The goal is to investigate the capabilities of Large Language Models (LLMs) in generating and correcting planning domain models and executing complex cognitive tasks, specifically focusing on planning and reasoning. Although traditionally used for language processing based on probabilistic retrieval methods, LLMs such as GPT-3 have shown unexpected linguistic behaviors, raising questions about their potential in more structured cognitive domains. The project will methodically evaluate LLM's performance in generating plans for a series of planning tasks derived from domains (e.g., those of the International Planning Competition) and in generating and correcting domain models.
Literature:
Kambhampati, Subbarao, et al. "LLMs Can't Plan, But Can Help Planning in LLM-Modulo Frameworks." arXiv preprint arXiv:2402.01817 (2024).
Kambhampati, S. (2024). Can large language models reason and plan?. Annals of the New York Academy of Sciences.
Huang, W., Abbeel, P., Pathak, D., & Mordatch, I. (2022, June). Language models as zero-shot planners: Extracting actionable knowledge for embodied agents. In International Conference on Machine Learning (pp. 9118-9147). PMLR.
Pallagani, V., Muppasani, B., Murugesan, K., Rossi, F., Srivastava, B., Horesh, L., ... & Loreggia, A. (2023). Understanding the capabilities of large language models for automated planning. arXiv preprint arXiv:2305.16151.
Supervisors: Prof. Dr. Marco Aiello / Dr. Ilche Georgievski / Ebaa Alnazer, M.Sc.
LLMs for Service-Oriented Computing
Explore how Large Language Models (LLMs), like GPT-4, can revolutionize automated service composition. This project focuses on the integration of LLMs into the service composition pipeline, transforming how adaptive information systems are constructed from loosely-coupled network services. The research is about delving into novel methods for unsupervised service composition, orchestration, and testing. Possible projects involve:
- Comparing LLMs for the task of service discovery, service composition, service orchestration
- Composition code generation with LLMs and their deployment
- Composition testing and validation
Literature:
Aiello, M., & Georgievski, I. (2023). Service composition in the ChatGPT era. Service Oriented Computing and Applications, 17(4), 233-238.
Pesl, R. D., Stötzner, M., Georgievski, I., & Aiello, M. (2023, November). Uncovering LLMs for Service-Composition: Challenges and Opportunities. In International Conference on Service-Oriented Computing (pp. 39-48). Singapore: Springer Nature Singapore.
Wolfram, S.: What Is ChatGPT Doing ... and Why Does It Work? Wolfram Research, Incorporated, Champaign, Illinois (2023)Bubeck, S., Chandrasekaran, V., Eldan, R., Gehrke, J., Horvitz, E., Kamar, E., Lee, P., Lee, Y.T., Li, Y., Lundberg, S., Nori, H., Palangi, H., Ribeiro, M.T., Zhang, Y.: Sparks of Artificial General Intelligence: Early experiments with GPT- 4. Technical Report 2303.12712, arXiv (2023)
Meyer, B.: What do ChatGPT and AI-based automatic program generation mean for the future of software. BLOG@Communications of the ACM 12 (2022)
Supervisors: Prof. Dr. Marco Aiello / Dr. Ilche Georgievski / Robin Pesl, M.Sc.
HTN Planning for Electric Vehicles- Knowledge Acquisition and Modelling
All these complexities call for the need to automate the planning process, a task that is powerfully handled by Artificial Intelligence (AI) planning, which is a subarea of Artificial Intelligence (AI). This subarea investigates the process of computing a course of action that satisfies a given user goal [5]. In the area of AI planning, Hierarchical Task Network (HTN) planning is well-known for its suitability to efficiently solve planning problems in real-world domains [6].
The aim of this project is to model the domain of EVs for HTN planning within the framework
proposed in [3]. The project includes the following topics:
- Defining the problem of planning the driving task for EVs as an HTN planning problem
- Acquiring knowledge about the domain of EVs in a systematic way (knowledge acquisition)
- Defining the requirements of the EVs domain
- Defining the entities and tasks that are part of this domain
- Defining the sources of uncertainty that exist in this domain and their effect on the variability of action costs
- Modelling the domain of EVs using a standard syntax, such as HPDL, by mapping the defined entities, tasks, and variable costs to HTN planning constructs
- Adapting an existing HTN planning approach to solve planning problems in this domain optimally while taking different risk attitudes into account
- Evaluating the proposed approach
Keywords: HTN planning, Electric vehicles, risk, uncertainty
Literature
[1] J. A. Sanguesa, V. Torres-Sanz, P. Garrido, F. J. Martinez, and J. M. Marquez-Barja, “A review on electric vehicles: Technologies and challenges,” Smart Cities, vol. 4, no. 1, pp. 372–404, 2021.
[2] J. Eisner, S. Funke, and S. Storandt, “Optimal route planning for electric vehicles in large networks,” in Twenty-Fifth AAAI Conference on Artificial Intelligence, 2011.
[3] E. Alnazer, I. Georgievski, and M. Aiello, “Risk Awareness in HTN Planning,” arXiv preprint arXiv:2204.10669, 2022.
[4] K. Valogianni, W. Ketter, and J. Collins, “Smart charging of electric vehicles using reinforcement learning,” in Workshops at the Twenty-Seventh AAAI Conference on Artificial Intelligence, 2013.
[5] M. Ghallab, D. Nau, and P. Traverso, Automated Planning: theory and practice. Elsevier, 2004.
[6] I. Georgievski and M. Aiello, “HTN planning: Overview, comparison, and beyond,” Artificial Intelligence, vol. 222, pp. 124–156, 2015.
Integrated Framework for Risk-Aware HTN Planning with Uncertainty-Aware Risk Detection
Planning driving tasks for autonomous vehicles (AVs) involves two main challenges: identifying risks in uncertain environments and incorporating those risks, along with risk preferences, into the planning process. While there has been progress in tackling these challenges, the solutions often address them separately.
One approach, developed by Puphal et al. (2019), focuses on detecting risks in dynamic environments using a probabilistic framework [2]. This method considers both aleatoric (uncertainties from the environment) and epistemic (uncertainties from the model) uncertainties to identify high-risk areas. Another approach, proposed by Alnazer et al. (2022), introduces a risk-aware hierarchical task network (HTN) planning framework that incorporates risk and risk attitudes in HTN planning constructs and planning process [4].
In the proposed project, the idea is to combine these approaches, which can offer a powerful solution. In particular, the uncertainty-aware risk detection method helps by providing accurate, real-time information about risks in the environment. This information serves as a key input for the HTN planning process, ensuring that plans are based on the latest and most reliable understanding of the situation.
The project is conducted in collaboration with the Honda Research Institute (HRI).
Keywords: HTN planning, risk-awareness, risk detection, uncertainty, autonomous vehicles
Literature
[1] E. Alnazer, I. Georgievski, N. Prakash, and M. Aiello, A role for HTN planning in increasing trust in autonomous driving, in IEEE International Smart Cities Conference (ISC2). IEEE, 2022, pp. 1–7.
[2] T. Puphal, M. Probst, and J. Eggert, Probabilistic uncertainty-aware risk spot detector for naturalistic driving, IEEE Transactions on Intelligent Vehicles, vol. 4, no. 3, pp. 406–415, 2019.
[3] A.-E. Taha and N. AbuAli, Route planning considerations for autonomous vehicles, IEEE Communications Magazine, vol. 56, no. 10, pp. 78–84, 2018.
[4] E. Alnazer, I. Georgievski, and M. Aiello, Risk Awareness in HTN Planning, arXiv preprint arXiv:2204.10669, 2022.
[5] M. Khonji, J. Dias, R. Alyassi, F. Almaskari, and L. Seneviratne, A risk-aware architecture for autonomous vehicle operation under uncertainty, in International Symposium on Safety, Security, and Rescue Robotics (SSRR). IEEE, 2020, pp. 311–317.
[6] I. Georgievski and M. Aiello, HTN planning: Overview, comparison, and beyond, Artificial Intelligence, vol. 222, pp. 124–156, 2015.
[7] T. Puphal, M. Probst, Y. Li, Y. Sakamoto, and J. Eggert, Optimization of velocity ramps with survival analysis for intersection merge-ins, in IEEE Intelligent Vehicles Symposium (IV), 2018, pp. 1704–1710.
[8] R. Wenzel, M. Probst, T. Puphal, T. H. Weisswange, and J. Eggert, Asymmetry-based behaviour planning for cooperation at shared traffic spaces, in 2021 IEEE Intelligent Vehicles Symposium (IV), 2021, pp. 1008–1015.
[9] F. Damerow, T. Puphal, Y. Li, and J. Eggert, Risk-based driver assistance for approaching intersections of limited visibility, in IEEE International Conference on Vehicular Electronics and Safety (ICVES), 2017, pp. 178–184.
Supervisor: Ebaa Alnazer, M.Sc., Dr. Ilche Georgievski ,Prof. Dr. Marco Aiello
Contact
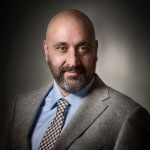
Marco Aiello
Prof. Dr.Head of Department
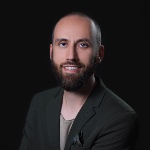
Ilche Georgievski
Dr.Akademischer Rat and Head of Division Planning, Learning, and Intelligent Systems
[Image: Ilche Georgievski]
Elisabeth Ibach
Secretary